Joining the dots: How AI and drones are transforming emergencies
In New York in 1997 Deep Blue, a chess-playing computer, made headlines after beating the then world chess champion Garry Kasparov — to whom it had lost a year earlier.
The computer's victory fuelled heated debates about ethics and human limits, but also inspired futuristic imaginings of robots taking over the world.
Human components — such as labour, regulation and development — are both the making and undoing of innovations. We should proceed with caution, though, to avoid the doomsday scenario: machines turning against humanity.
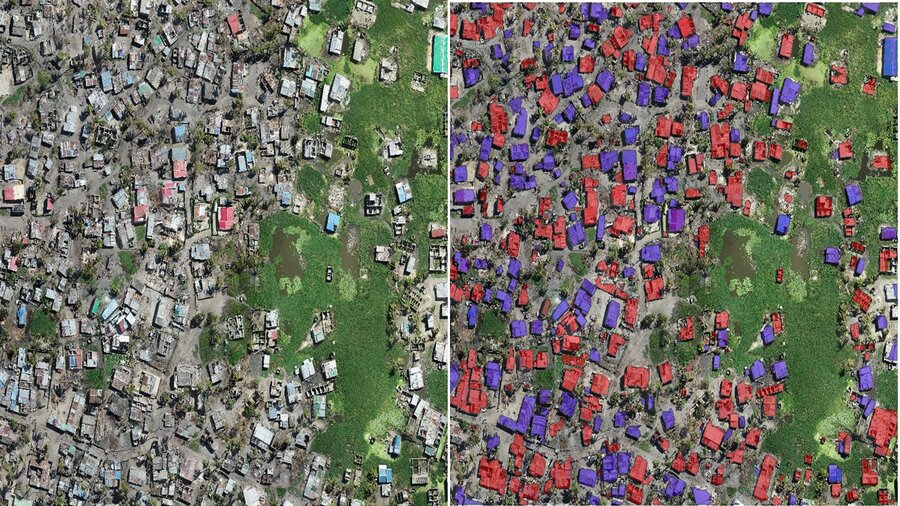
A long-held misconception about computers is that they will make human labour redundant. Machines have some obvious advantages over humans: they can be counted on for continual productivity. The so-called "third industrial revolution" which commenced at the start of the 1970s, brought a degree of automation to manufacturing.
Saving time by outsourcing manual labour led to worries that machines could replace humans in more tasks than originally in. We're now navigating the digital, and ethical, landscape of what some are calling the "fourth industrial revolution". Experts agree that the complexity of artificial intelligence, including machine learning, fails to mimic the exact neural processing of the human brain.
What do humans have that machines still lack — abstract forms of intelligence like empathy, common sense, and knowledge which function in tandem across different areas.
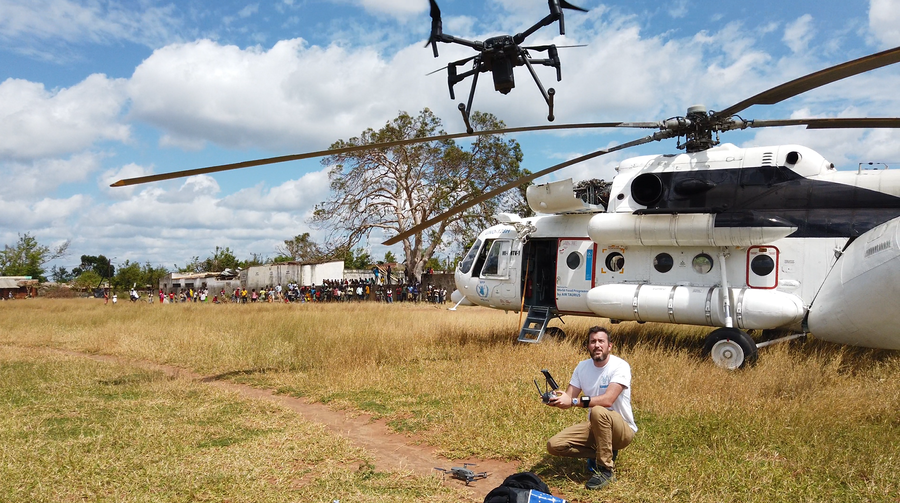
Such features are still difficult to translate into an algorithm and so cannot be transferred to a machine. This eliminates the possibility of, well, eliminating human input from the machine-learning equation.
"Machine learning simplifies or speeds up what humans can do," says Marco Codastefano, a data scientist with the World Food Programme (WFP). AI makes humanitarian work faster and more smoothly. Coupled with human ingenuity, ethics and good judgement, the possibilities for AI applications in the humanitarian field are many.
Want a better emergency response? Add AI to drones
When used responsibly, technology improves everyday lives, but it can also benefit those affected by disasters and crises by supporting humanitarian organizations. After cyclones Idai and Kenneth tore across Mozambique in March and April last year, AI and drones were officially used for the first time in a humanitarian emergency by WFP.
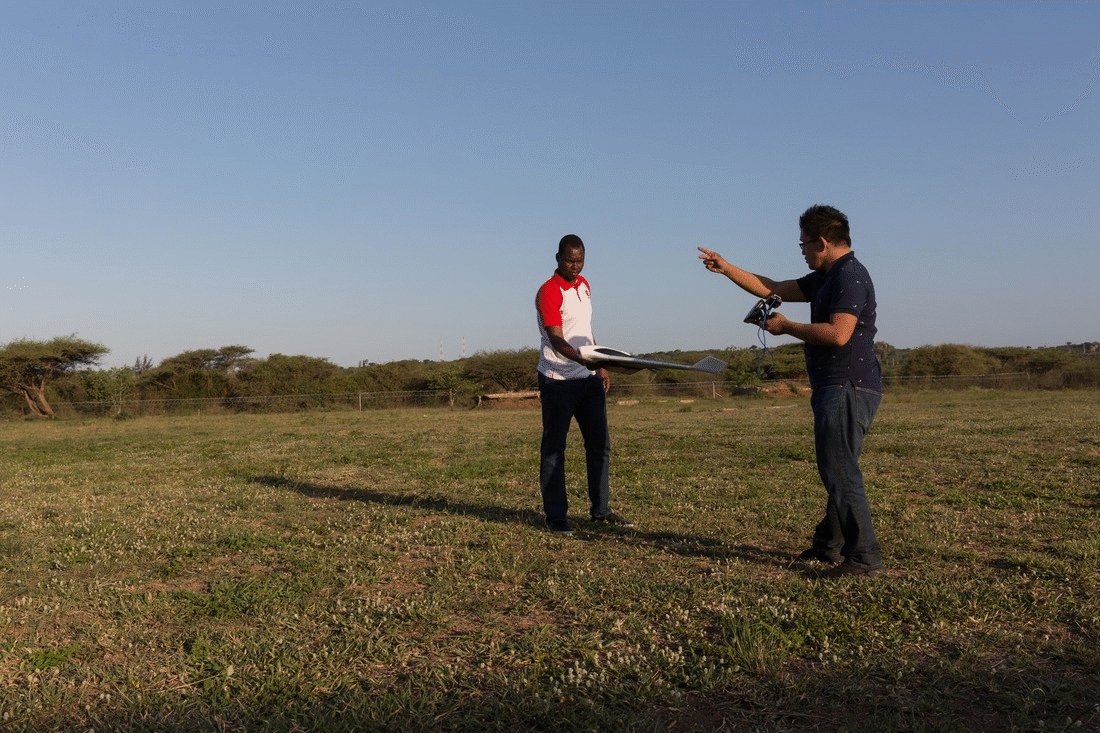
The wins were enormous. In any emergency, information is key. "What we can do is to speed up our response to minimize the impact and to help people rebuild their lives," says Codastefano.
Drones offer a relatively cheap way of obtaining the data needed to make fast decisions when time is running out. "One of the crucial tasks in an emergency is to spread the knowledge to local responders," he says.
Thousands of drone images were collected and stitched into a quilt of data-rich maps, laying out the information that government and relief organizations needed for critical decisions: where and how to deliver aid in the Sofala province of central Mozambique and, later, in Cabo Delgado, in the north of the country.
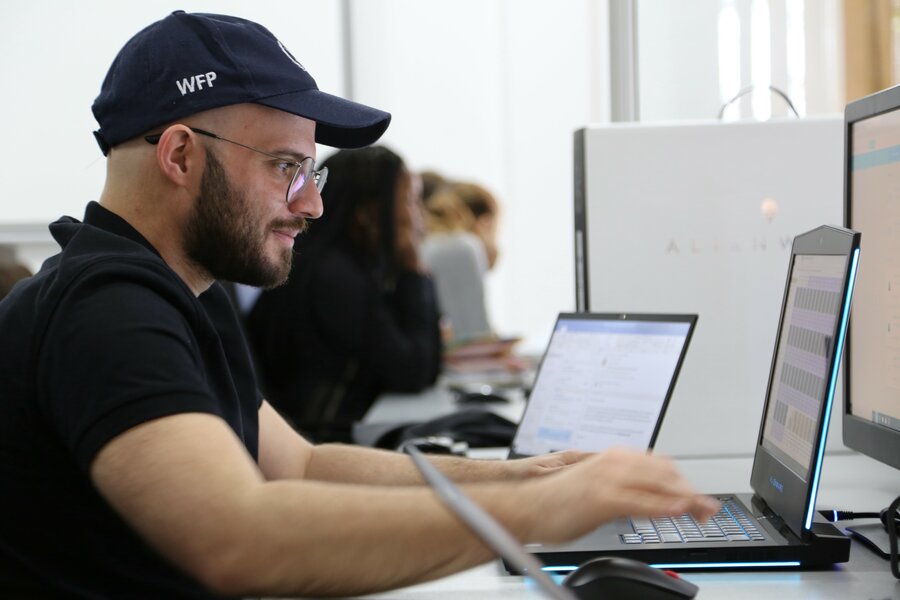
Regardless of how good the images were (compared to satellite images which were either lower resolution or not available at all due to continued cloud coverage) it still took days to analyze them.
This is where AI entered the picture. DEEP (Digital Engine for Emergency Photo-analysis), an in-house WFP solution, reduced the analysis time from weeks to hours. Developed by Codastefano, DEEP is based on open-source applications. This means its source code is visible to programmers to inspect, change or enhance. Field-tested in Mozambique, it's proved to be a game-changer.
Always learning
"The idea [was] to develop an algorithm that allows us, from the mapping we have done with drones, to quickly quantify the number of houses that exist in a specific area and quantify the houses that were partially and completely destroyed," says Antonio Beleza, deputy director at the National Institute of Disaster Management (INGC) in Mozambique.
Drones, combined with AI, gave the emergency response an instantaneous and intelligent bird's-eye view of structural damage. Now governments, donors and local communities can get a fast and complete picture of damaged infrastructure, predict the cost of repairs, estimate how many people require emergency housing and mobilize accordingly.
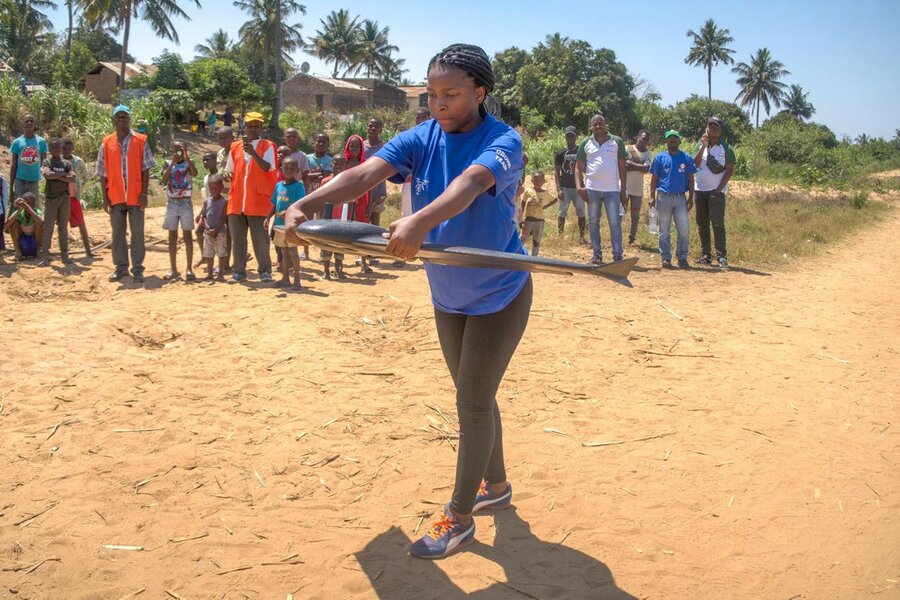
The humanitarians who responded in Mozambique showed a natural interest in learning and for exploring how technology can help us reach further.
"Once the emergency phase is over … we want to start preparing for the next rainy season", says Beleza. The INGC pilots trained by WFP in 2017 and 2018, were among the first-responders to cyclones Idai and Kenneth. They eagerly field-tested DEEP in June, and three months later were already mapping the Licungo river basin using fixed-wing drones as part of their preparedness efforts, ahead of flood season. The pilots are always on standby for the next disaster, ready to map and analyze at a moment's notice.
And all this learning is not just happening inside human brains; like hiking trails in a forest, neural pathways in humans and machines become better defined the more they are used.
"In Mozambique, we trained [the computer] to detect buildings. In the next emergency we can teach the model to detect another object," Codastefano says. Objects can be anything from infrastructure like bridges and roads to more abstract forms like standing water. There are plans to use more sophisticated cameras on drones, opening the door for inflight data analysis.
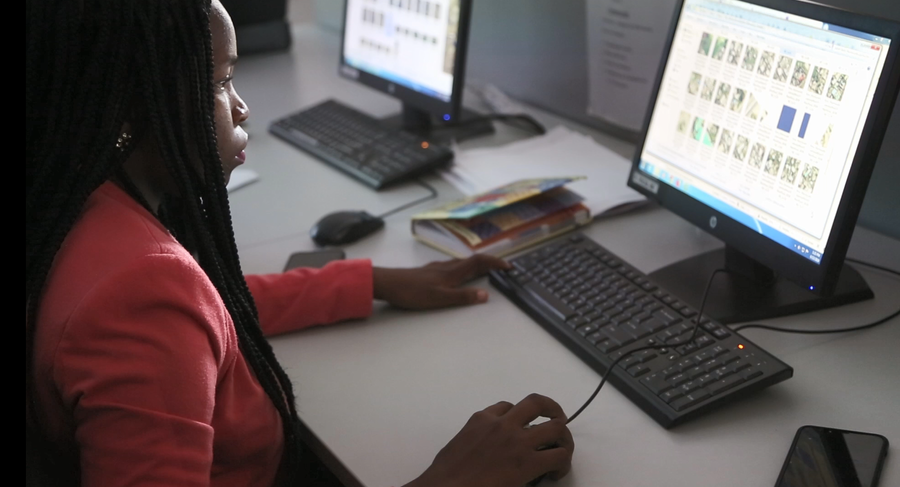
With the success of DEEP, new ideas are emerging about how to get the most from the blossoming symbiotic relationship between drones, AI and machine learning. Drones are best known for their high-resolution imagery, but they are also "a mobile sensor station and a data source," says Codastefano. When measuring devices are attached to them, drones can capture information invisible to the naked eye, and when AI is added to this mix, humanitarians can extract data for informed decision-making. WFP is looking to use this technology for crop-type mapping or analyzing the health of plants before they suffer visible damage.
Checks and balances
An open letter on AI, signed by Stephen Hawking, Elon Musk and other experts, was released in 2015 asking researchers to carefully consider the societal impact of AI, acknowledging its potential for good — while cautioning about the risks of "runaway technology" if it is not properly managed.
They also laid out specific areas of interdisciplinary research to match technological development with society's evolving needs, for example by addressing issues such as labour market changes, the concentration of power, and discrimination based on parameters such as gender, ethnicity and so on.
Currently, research and development in AI is dominated by men, with women representing less than 15 percent of the workforce, according to the World Economic Forum, 2019. Similar forms of discrimination can be found in machine learning algorithms related to race, as some face recognition apps struggle, for example, to detect darker shades of skin tone.
Such skewed representation can lead to further reinforcement of gender and racial stereotypes across various fields — from insurance companies judging the "creditworthiness" of a prospective client to employers deciding on a job applicant— that sometimes use AI to prevent human biases and make fairer decisions. Biased human perceptions inform the data which, in turn, informs AI.
Checks and balances need to be put in place to address a range of issues stemming from the deepening relationship between machines and humans: ranging from safety and security to ethics and data protection. WFP, equipped with decades of experience in aviation, logistics and telecommunications, will help to blueprint the social and technological architecture for humanitarian drones and AI.